Condition Monitoring of a Reciprocating Compressor
Current maintenance for large machines works by replacing parts on time intervals instead of the actual health of the part. This is inefficient because it wastes parts that have a remaining useful life and allows parts that no longer work properly to remain in the machines. Condition monitoring tries to estimate the actual health of the parts while the machines are running and the future health of the parts.
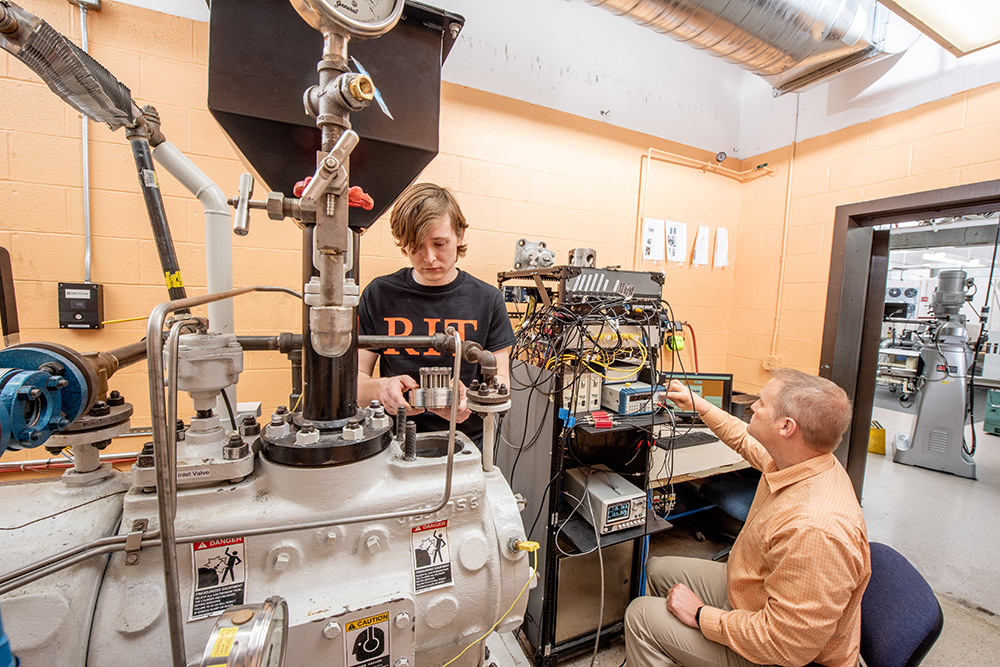
Current maintenance for large machines works by replacing parts on time intervals instead of the actual health of the part. This is inefficient because it wastes parts that have a remaining useful life and allows parts that no longer work properly to remain in the machines. Condition monitoring tries to estimate the actual health of the parts while the machines are running and the future health of the parts. It tries to schedule maintenance around the planned down time of the machines to minimize the time the machines are not running during production time.
Under the advisory of Dr. Jason Kolodziej, condition monitoring techniques have been studied and applied to a reciprocating compressor. Reciprocating compressors can compress various gases and are used in the oil and gas industry and many other fields. They are reliable but have a high maintenance cost with roughly a third of the maintenance costs relating to the compressor valves. Due to this, the research has been focused on improving condition monitoring of valve health.
The research starts with placing faulty components in the valves to collect signal data used to train many different algorithms to detect faulty valves. The signal data includes the pressure inside the compressor and the vibration on the outside of the valves. The pressure data is a good indication that a valve has become bad as it directly changes the pressure. However, vibration data can indicate that a problem is about to occur before a specific problem arises but can be more easily affected by outside factors such as vibrations from the environment.
The other part of the research develops algorithms to process and analyze the signal to determine the health of the valves. The algorithms range from statistical classifiers that create simple boundaries to deep learning models that can create complex boundaries. The statistical classifiers require the signals to be preprocessed and are good at detecting valve faults with high classification accuracy. Deep learning models can take the raw signals and achieve a higher classification accuracy but requires more data and more computer power.