Imaging Science Thesis Defense: On the Optimization of Sensors for the Remote Sensing of Crop Yield
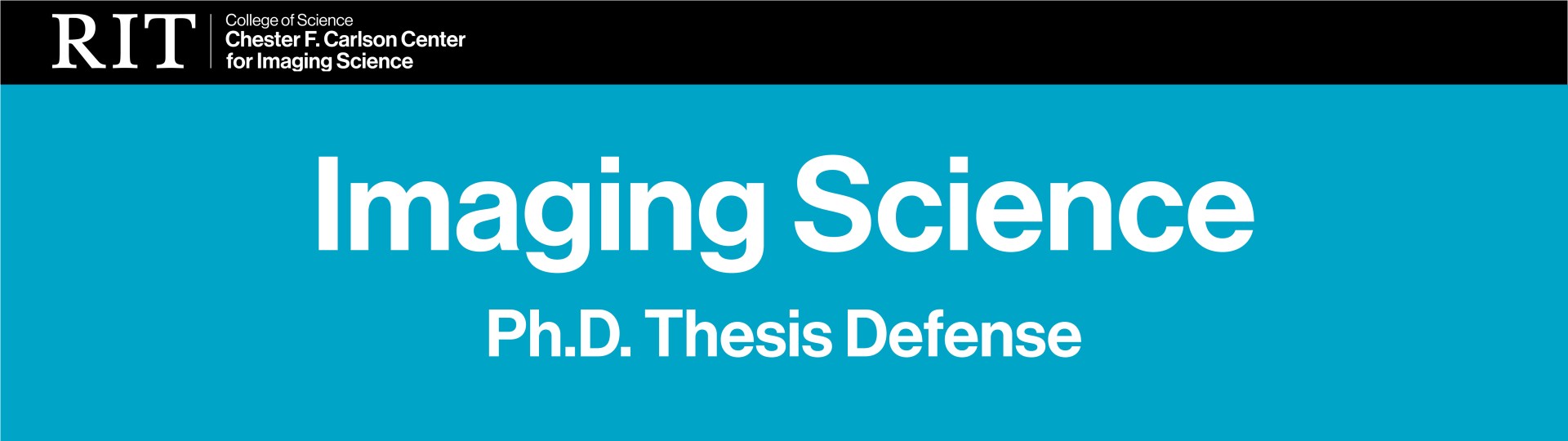
Imaging Science Thesis Defense
On the Optimization of Sensors for the Remote Sensing of Crop Yield
Nate Burglewski
Imaging Science
Rochester Institute of Technology
Register for Zoom Link Here
Abstract:
Remote sensing has long been a part of precision agriculture research, with products related to spatially-explicit, within-field management of yield, disease, and harvest scheduling, to name a few application areas that are of interest to industry. These spaceborne, airborne, and in-situ sensors are used to monitor crop health throughout the growing season, inform management decisions for farmers, and dictate logistical or planning operations. Crop yield forecasting, specifically, comes in many forms, but recently machine learning algorithms have gained prominence, at the cost of more traditional parametric regression models. While much work has been accomplished towards grain yield estimation using remote sensing data, silage yield forecasting has been less investigated. Furthermore, to date no sensor has been designed to optimize the spatial-spectral trade space that is relevant to yield estimation. We thus sought to identify the ideal spectral and spatial sampling parameters for a sensor to be used for this purpose. We first set out to identify a yield estimation algorithm which was favorable for both grain and silage yield, finding that support vector regression performed best at both field-level and regional yield estimates for corn grain. We then leveraged three separate spectral band selection algorithms to quantitatively assess the most important spectral content found in our high spatial resolution, high spectral dimensional imaging spectroscopy data. These data were resampled both spectrally and spatially to determine the interplay of spatial resolution and spectral content on corn grain and silage yield estimation results. Corn grain yield estimation accuracies ranged from 7.02% mean absolute percentage error (MAPE) (root mean square error [RMSE] = 0.332 Mg/ha) at 0.06 m GSD to 11.45% MAPE (RMSE = 0.435 Mg/ha) at 30 m GSD using data collected at the same five band multispectral sampling configuration during the R4 (dough) growth stage. We found that this favorability extended to silage yield estimation at the same growth stage, with MAPE ranging from 2.22-2.84% MAPE (0.62-0.68 Mg/ha) from 0.06-30 m GSD.
We thus were able to identify the spectral features which were ideal for this purpose using high resolution imaging spectroscopy data, while also investigating the changes in estimation accuracies as a function of spectral content and spatial resolution. Ultimately, the most favorable spectral configuration was multispectral, containing blue (450 nm), green (550 nm), red (670), and three near-infrared spectral bands (770, 830, and 920 nm). This arguably is an ideal outcome, as sampling spectral content along these bandpasses greatly reduces dimensionality resulting from imaging spectroscopy data, while preserving the most important features for a machine learning regression algorithm to exploit. This spectral configuration was found to give the most accurate regression results, specifically centered at 8 -16 m GSDs for corn silage yield, even though there were changes in accuracy across a wide range of spatial resolutions. We attributed this result to matching the semivariance range of the yield data, which in turn generated favorable modeling conditions for the support vector regression estimation. For corn grain, the average semivariance range was greater than our largest sampling distance, so in general the higher spatial resolutions below 1 m GSD were most accurate. These findings inform sensor engineers of the optimal spectral sampling configuration for an idealized sensor for use in corn grain or silage yield estimation at any spatial scale. Future work should address the underlying yield drivers behind these selections to further isolate the spectral content correlated to crop health and status metrics like nitrogen content, heat stress, water stress, and structural parameters. These are all correlated with yield and thus important to stakeholders, leading to potential mitigation via management techniques to improve yield projections.
Intended Audience: All are Welcome!
To request an interpreter, please visit myaccess.rit.edu
Event Snapshot
When and Where
Who
This is an RIT Only Event
Interpreter Requested?
No