Imaging Science Seminar Learning with Weak Supervision - Algorithms and Applications
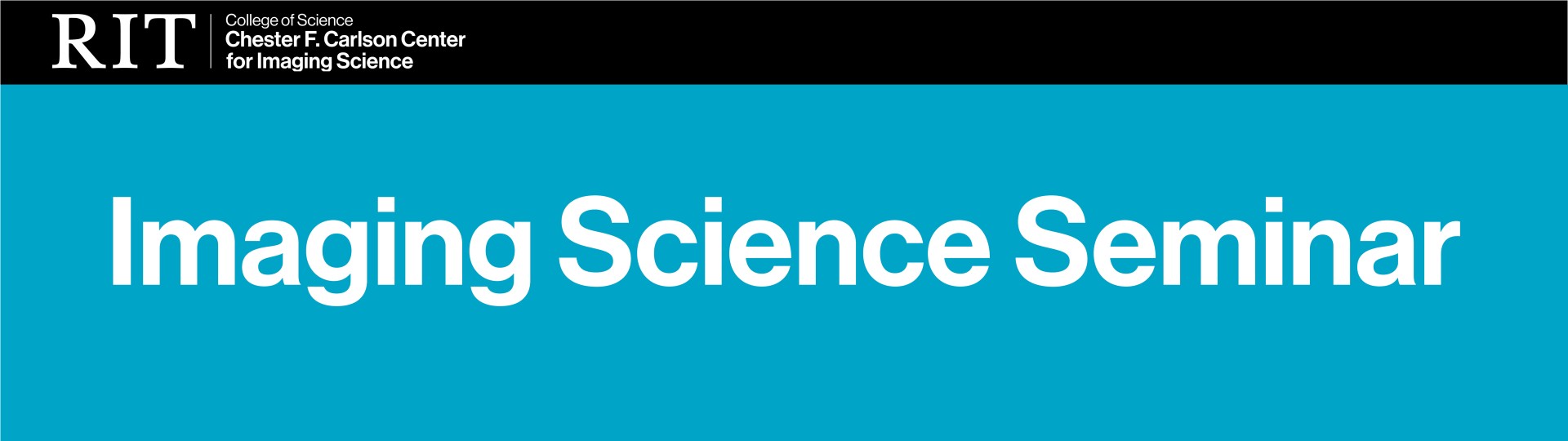
Imaging Science Seminar
Learning with Weak Supervision: Algorithms and Applications
Dr. Shayok Chakraborty
Assistant Professor
Computer Science
Florida State University
Annotating the enormous amounts of data that is available today to induce a machine learning model has remained a fundamental challenge. Thus, developing intelligent machine learning algorithms under the constraint of weak manual supervision is a problem of immense practical importance.
Abstract:
The widespread deployment of inexpensive sensors has resulted in the generation of enormous amounts of digital data in today’s world. This has expanded the possibilities of solving real-world problems using computational learning frameworks; however, annotating the data (with class labels) to induce a machine learning model has remained a fundamental challenge. Thus, developing intelligent machine learning algorithms under the constraint of weak manual supervision is a problem of immense practical importance. Active learning and domain adaptation (or transfer learning) are two methods to address this problem. Active learning algorithms automatically select the salient and exemplar samples from large amounts of unlabeled data; this tremendously reduces human annotation effort as only a few samples selected by the algorithm need to be annotated manually. Domain adaptation algorithms leverage abundant labeled data in a source domain to develop a model for a related target domain, where labeled data is scarce, under the constraint of a probability distribution difference between the two domains. These two learning paradigms are even more important for training deep neural networks, which have demonstrated commendable empirical performance, but require a large amount of labeled data. In this talk, I will present an overview of several research problems in weakly supervised learning, that I am currently exploring in my research at FSU.
Speaker Bio:
Dr. Shayok Chakraborty is an Assistant Professor in Computer Science at Florida State University. Prior to joining FSU, Dr. Chakraborty held a research faculty position at Arizona State University, where he was an Associate Director of the Center for Cognitive Ubiquitous Computing (CUbiC) laboratory. He received his PhD in Computer Science from Arizona State University in 2013. He has worked as a post-doctoral researcher at Intel Labs, Oregon and in the Electrical and Computer Engineering department at Carnegie Mellon University. He also had a collaboration with the machine learning department at Microsoft Research, Redmond. Dr. Chakraborty’s research interests include machine learning, computer vision and assistive technology. He has published his research in premier conferences and journals in these areas, including AAAI, KDD, CVPR, TPAMI and TNNLS among others. He also extensively serves as an area chair, as a program committee member and as a reviewer of these conferences and journals. His paper on person-centered multimedia computing received the 2017 IEEE Multimedia Best Department Article Award. He is also the recipient of the 2019 Amazon Research Award and the 2019 AWS Machine Learning Research Award. Together with his collaborators, Dr. Chakraborty received the Best Interdisciplinary Research Award at the Aging and Health Informatics Conference 2021. He has presented tutorials on his research on active learning at IEEE ICME 2013, IEEE WACV 2019 and ACM Multimedia 2020. He is a senior IEEE member.
Intended Audience:
Undergraduates, graduates, and experts. Those with interest in the topic.
Event Snapshot
When and Where
Who
Open to the Public
Interpreter Requested?
No