Imaging Science Thesis Defense: Morphology Classification of High Redshift Galaxies from JWST NIRCam Images Using Unsupervised Machine Learning
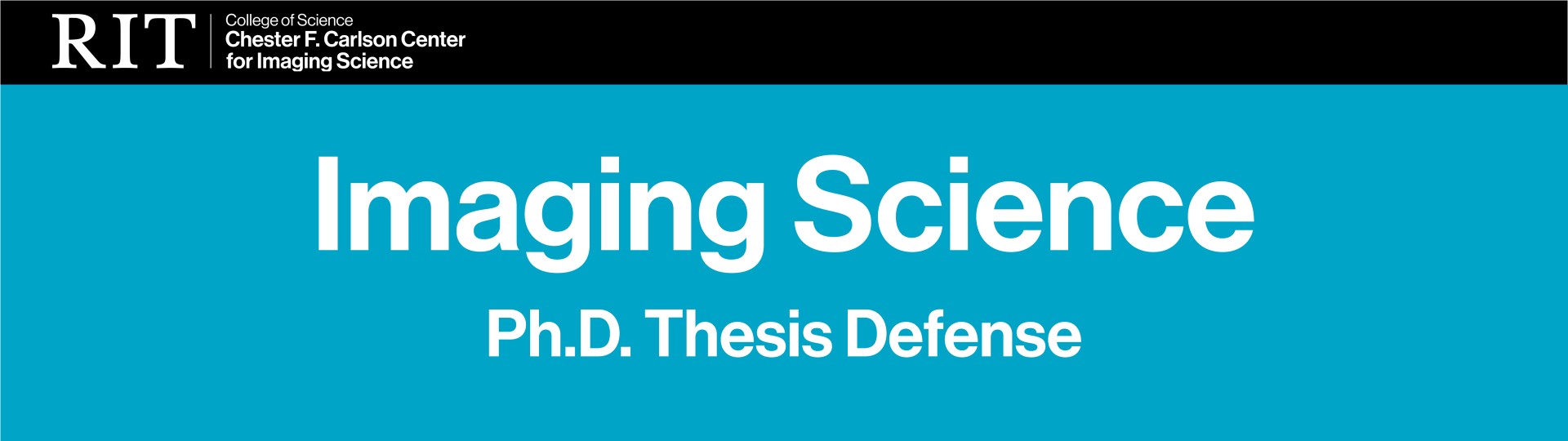
Imaging Science Thesis Defense
Morphology Classification of High Redshift Galaxies from JWST NIRCam Images Using Unsupervised Machine Learning
James Liu
Imaging Science
Rochester Institute of Technology
Register for Zoom Link Here
Abstract:
The James Webb Space Telescope (JWST) has revolutionized our ability to observe high redshift (z > 2) galaxies, providing a glimpse into the early universe. Classification of these galaxies is crucial to advancing our understanding of galaxy and cosmic evolution. This work aims to develop a machine-based classification scheme for high redshift galaxies using JWST data. Given the immense volume of data generated by JWST, traditional classification methods are insufficient, necessitating an automated approach. We implement an unsupervised machine learning technique that combines a Vector-Quantized Variational Autoencoder (VQ-VAE) as a feature extractor and the K-means clustering algorithm as the classifier to group galaxies based on similar features. This method is applied to over 12,000 simulated galaxies based on the Cosmic Evolution Early Release Science (CEERS) survey and over 250,000 real galaxies from the COSMOS-Web survey to assess its effectiveness. Our model successfully extracts and clusters features from both datasets; however, the results indicate differences between using simulated and real data. The complexity in real JWST data posed challenges not encountered with simulated data, highlighting the need for further refinement in our approach. Efficient classification of high redshift galaxies is essential for mapping the evolutionary path of the universe. Our study contributes a novel classification scheme that incorporates high redshift galaxies, paving the way for more detailed and efficient scientific studies in galaxy evolution. This work highlights the importance of addressing the complexities of working with real astronomical data in developing a more robust model for machine-based classification.
Intended Audience:
All are Welcome!
To request an interpreter, please visit myaccess.rit.edu
Event Snapshot
When and Where
Who
This is an RIT Only Event
Interpreter Requested?
No