Two Researchers Create AR System for Teaching Robots New Skills
Xueting Wang and Dr. Yunbo Zhang have developed an advanced AR system that allows users to teach robots new tasks with just a few demonstrations, making human-robot interactions more intuitive and efficient.
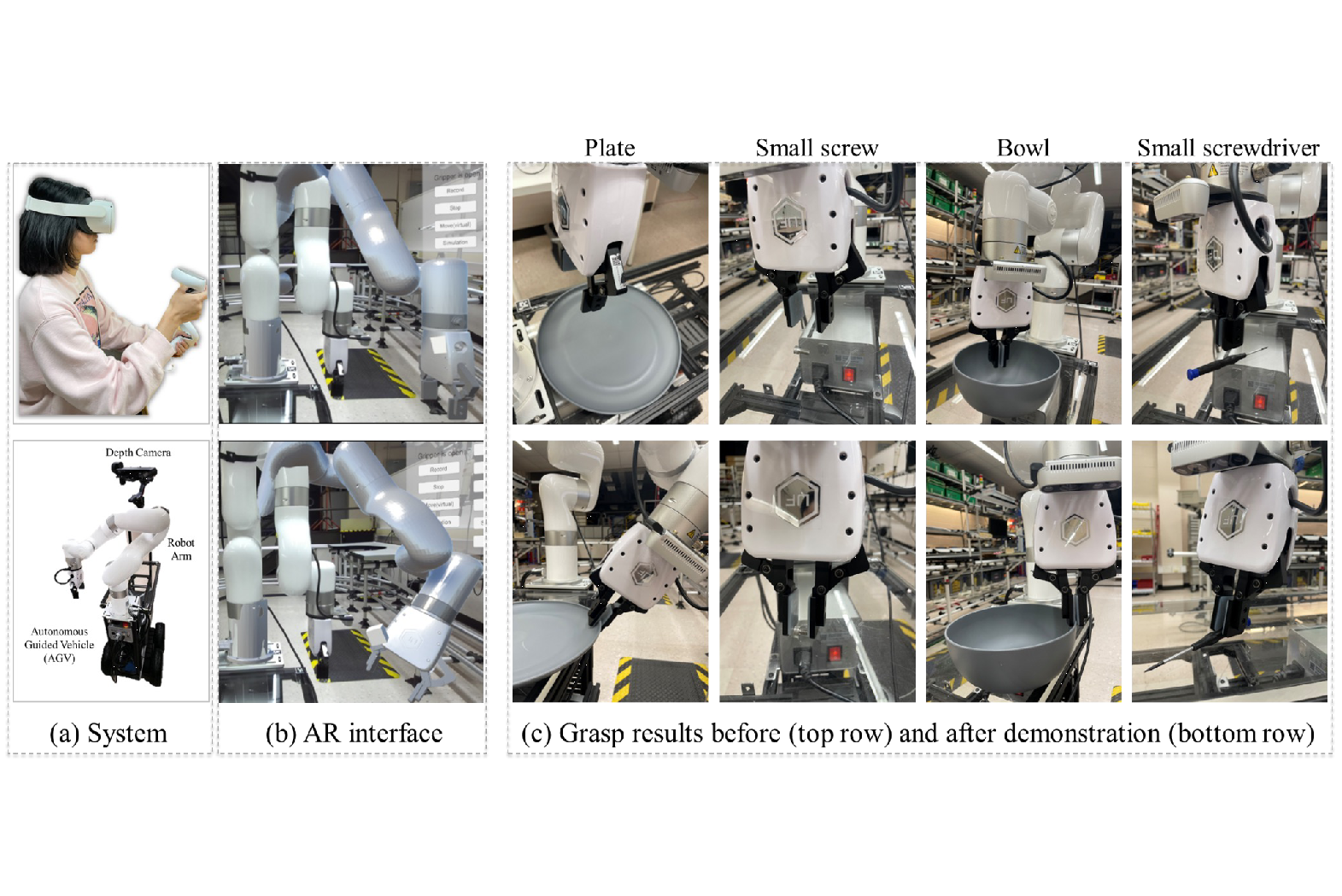
Under the supervision of Dr. Yunbo Zhang, the novel robot control and interaction system based on machine learning architecture has been developed by Xueting Wang, student in Mechanical and Industrial Engineering Ph.D. The proposed system enables humans to control the remote robot and teach it new skills within three demonstrations. This collaborative project involved both the Rochester Institute of Technology (RIT) and the humanoid robot startup Figure AI.
Training a robot to perform tasks presents significant challenges due to limitations in communication between the robot instructor and the robot itself. The existing barrier hinders then robot's ability to independently comprehend human instructions effectively. The project mainly develops an interactive system in the Augment Reality (AR) environment to enhance the communication between human and the robot and an intelligent robot learning framework to enable robot to capture the operating strategy from robot instructor.
The AR interactive system that has been developed has the primary objective of facilitating natural language communication between humans and robots, empowering the robot to actively articulate its requirements. Additionally, the intelligent robot learning framework is designed to acquire task-related skills, ranging from basic actions like grasping, as illustrated in figure 1, to more intricate tasks such as assembling a gear box, instructed by a human instructor during human-robot interaction. Traditionally, robot instructors are expected to possess substantial knowledge of robotics and experience in maneuvering 6-DoF robotic arms. However, this project aims to alleviate the prerequisite knowledge burden on robot instructors, offering an efficient solution for robots to assimilate complex tasks effectively.
The project main contributions include introducing an AR human-robot teleoperation framework, integrating large language models for natural language interaction and a user-friendly interface displaying future robot plans. Additionally, a robot learning framework was developed to efficiently extract crucial information from human-robot interactions, enabling effective execution of specific operation plans. Moreover, a comprehensive framework leveraging vision language models within the robot system was proposed, covering environment perception, targeted query generation, and precise operation plan formulation for enhanced robotic autonomy.