AIRG
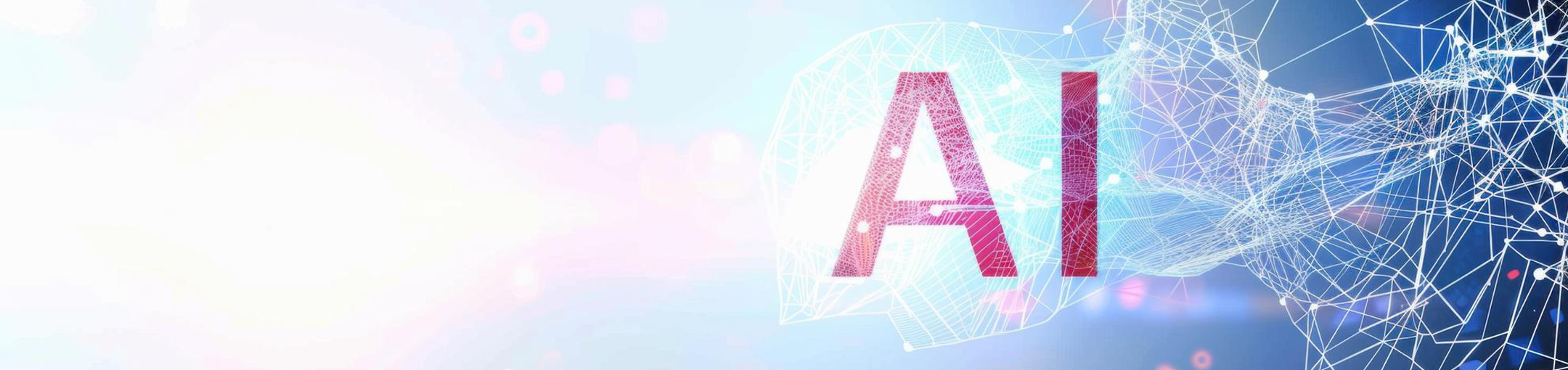

AI Research Group
Advancing innovation through cutting-edge research and collaboration
- RIT/
- RIT Dubai/
- About/
- Research Groups/
- AI Research Group
Artificial Intelligence Research Group
(AIRG)
At the AI Research Group (AIRG), we address fundamental challenges in AI research, with a core focus on healthcare, as well as mobility, cybersecurity, blockchain, communications, the metaverse, and data science. Our mission is to advance AI methodologies, create scalable solutions, and foster interdisciplinary innovation.
Goals of the AIRG
- Developing innovative AI-driven solutions to address complex challenges and optimize processes across various industries, focusing on healthcare.
- Building strong collaborations with academia to foster knowledge exchange and interdisciplinary research, ensuring cutting-edge advancements in AI.
- Attracting research grants to support innovative projects and expand the scope of our research across multiple sectors.
- Connecting with government and industry partners to align our AI research with real-world needs, facilitating the development of impactful solutions and driving innovation in key industries.
Faculty
Jinane Mounsef
Abdulla Ismail
Wesam Almobaideen
Ioannis Karamitsos
Assistant Professor of Data Analytics
Omar Abdul Latif
Ali Assi
Mehtab Khurshid
Healthcare
Early Cervical Cancer Detection
Researcher: Dr. Jinane Mounsef
Collaborators: MBRU and WAI
Cervical cancer ranks as a leading cause of mortality among women, with the conventional pap smear-based detection posing significant challenges due to its labor-intensive and time consuming nature. This study explores how Artificial Intelligence (AI) technology can be integrated into cervical cancer diagnostics to alleviate the workload of pathologists by streamlining and improving the detection process.
We used deep learning (DL) models, specifically VGG-9, VGG-16, Inception, and Resnet-50, to facilitate early identification of precancerous cervical cells from pathological slide images. Our research includes a detailed comparison of these models’ performances, highlighting the Inception model’s exceptional accuracy of 98% in early detection efforts. Finally, we propose Cerviscan, a comprehensive system for early cancer detection.
AI-Driven Objective Pain Measurement Using Biosensors
Researcher: Dr. Jinane Mounsef
Collaborator: MBRU
Accurately measuring pain has long been a challenge in medical diagnostics due to its subjective nature, often relying on patient self-reporting. This research explores the use of artificial intelligence (AI) to develop an objective, data-driven approach for pain assessment through the integration of biosensors. By analyzing physiological signals such as heart rate variability, skin conductance, facial expressions, and muscle activity, AI models can identify patterns that correlate with pain intensity. The proposed system leverages machine learning algorithms to process multimodal data from biosensors, providing real-time and personalized pain assessment. This AI-driven method offers the potential to improve pain management, enhance patient care, and reduce the reliance on subjective reporting, especially in cases where patients are unable to communicate effectively. The ultimate goal is to create a reliable, scalable solution that bridges the gap between subjective perception and objective measurement in clinical and remote healthcare settings.
Development of Advanced Smart Home Systems for Enhanced Elderly Healthcare through AI and IoT Integration
Researcher: Mehtab Khurshid
Mehtab's research is centered on the development of advanced Smart Home Systems, with a primary focus on enhancing healthcare for the elderly, though the scope is intentionally broad to encompass other demographics. The objective is to alleviate the burden on healthcare facilities by enabling effective chronic disease monitoring within the comfort of individuals' homes. This research involves constructing a robust Reference Model through a structured, multi-phase approach. The process includes the design and development of a domain-specific language, sophisticated workflow orchestration frameworks, and the seamless integration of IoT devices and relevant services using Artificial Intelligence and Machine Learning models. These components are engineered to operate across multiple layers, culminating in a fully interactive and cohesive system that is both technically sound and practically applicable.
Energy
Two-way Load Flow Analysis using Newton-Raphson and Neural Network Methods in Smart Grids
Researcher: Dr. Abdulla Ismail
This research presents a study of the optimal power flow for networked microgrids with multiple renewable energy sources (PV panels and wind turbines), storage systems, generators, and load. The OPF problem is performed using a conventional method and an Artificial Intelligence method. In this research, we investigated the performance of MGs system with renewable energy integration with focus on power flow studies.
The calculation of the power flow is based on the well-known Newton-Raphson method and Neural Network method. The power flow calculation aims to evaluate the grid performance parameters such as voltage bus magnitude, angle, real and reactive power flow in the system transmission lines under given load conditions. The standard test system used was a benchmark test system for Networked MGs with four MGs and 40 buses. The data for the entire system has been chosen as per the IEEE Standard 1547-2018. The results showed minimum losses and higher efficiency when performing OPF using NN than the Newton-Raphson method. The efficiency of the power system for the networked MG is 99.3% using Neural Network and 97% using the Newton-Raphson method. The Neural Network method, which mimics how the human brain works based on AI technologies, gave the best results and better efficiency in both cases (Battery as Load/Battery as Source) than the conventional method.
Modelling and Load Frequency Control (LFC) Design of Microgrid Frame-worked As Technological Norm to Multi Energy System (MES)
Researcher: Dr. Abdulla Ismail
In today's world, the trend of using renewable energy sources as an alternative energy source is becoming more and more common due to various driving factors, such as energy scarcity (fossil fuel depletion, etc.) and environmental issues (carbon footprint). This trend is leading to a steady increase in the penetration of renewable energy sources (RES) through microgrids and DER, which consist of RES and are integrated into the power grid. As a result, the power system is becoming more complex and operations are subject to a less structured and uncertain environment. The uncertainties in the power system and the inherent high fluctuations of RES usually make conventional controllers less effective in providing adequate load frequency control (LFC) for diverse and wide operating conditions. In addition, the virtual inertia system, which has been thoroughly studied and verified in this work, cannot maintain and stabilize the frequency deviation within an acceptable range and cannot fully be relied on reducing the uncertainty in operation during disturbances due to the fluctuation of RES and abrupt load changes. Accordingly, detailed and continuously improved control techniques are required for stability and operation uncertainty reduction reasons. Therefore, an advanced control technique based on LQG (LQR with Kalman filter for state estimation) for an isolated microgrid structured as a multi-energy system (MES) is presented in this thesis. The introduction of MES into the microgrid structure helps to achieve a high level of synergy among the different subsystems/units and maximize the use of RES within the system, thereby contributing to frequency stability in MG. The performance of the proposed advanced controller is compared with conventional proportional-integral-derivative (PID) – PSO tuned controllers and interval type 1 (IT1) fuzzy controller design methods. The result shows that the LQG controller has better or lower settling time, improves the dynamic responses, and is highly resilient to severe load or active power disturbances for the subject MG.
Cybersecurity
Unsupervised Instance Matching in Knowledge Graphs Using GAN-Based Language Translation
Researcher: Dr. Wesam Almobaideen
The Instance Matching technique involves identifying instances across different Knowledge Graphs (KGs) that refer to the same real-world entity. This process typically requires significant time and effort, especially with large KGs.
Traditionally, this problem is addressed in a supervised manner, where a set of corresponding instance pairs across different KGs is available. However, in real-world applications involving billions of instances, such sets are often incomplete, adversely affecting the recall score. To address this challenge, our proposal explores an unsupervised approach by representing the instance matching problem as a language translation task. This is achieved through the use of a shared-latent space based on Generative Adversarial Networks (GANs), which allows for effective matching even in the absence of complete paired instances.
Machine Learning-Based Intrusion Detection System: A Novel Sinh Cosh Optimizer with XGBoost Approach for Attack Detection in Metaverse
Researcher: Dr. Abdulla Ismail
Networks that combine metaverse and Internet of Things (IoT) technologies are vulnerable to various privacy and security risks. This makes it necessary to develop intrusion detection systems as a very effective way to immediately detect these forms of attacks. The convergence of the metaverse with the IoT will create increasingly diverse virtual and intelligent networks. Integrating IoT networks into the metaverse will facilitate stronger connections between the virtual and physical world by enabling:
- Real-time data processing
- Retrieval
- iInspection
Due to the interactive nature of the metaverse and the numerous human interactions that occur in virtual worlds, a large amount of data is generated which leads to computational complexity in building an intrusion detection system. The proposed work presents a novel model for intrusion detection system that relies on machine learning (ML) and optimization algorithms to address this problem. This model uses a combination of two techniques, namely:
- k-nearest neighbors (KNN)
to detect and classify attacks - Sinh Cosh optimizer (SCHO)
to identify the most important features and obtain the highest performance.
Its purpose is to effectively detect the bulk of attacks on Metaverse-IoT connections. The effectiveness of the proposed SCHO-XGBoost model is evaluated by using the widely recognized benchmark datasets:
- CIC-IDS2017
- CSE-CIC-IDS2018
- CICIoT2023
These datasets include a variety of IoT threats that have the potential to compromise IoT communications. Based on experimental evidence, the proposed model SCHO-XGBoost has proven effective in identifying 17 specific types of threats associated with Metaverse-IoT. It achieved the highest accuracy, recall, and f1-score rate of up to 99.9% on CSE-CIC-IDS2017, where it was compared with other benchmarked ML algorithms and proved superior to all algorithms. In addition, our SCHO-XGBoost model shows greater performance in accurately detecting attacks when compared to other models discussed in the existing literature.
Automated Detection of Cyber Attacks in Healthcare Systems: A Novel Scheme with Advanced Feature Extraction and Classification
Researcher: Dr. Abdulla Ismail
The growing incorporation of interconnected healthcare equipment, software, networks, and operating systems into the Internet of Medical Things (IoMT) poses a risk of security breaches. This is because the IoMT devices lack adequate safeguards against cyberattacks. To address this issue, this article presents a proposed framework for detecting anomalies and cyberattacks. The proposed model employs the 1) K-nearest neighbors (KNN) algorithm for classification, while 2) utilizing long-short term memory (LSTM) for feature extraction, and 3) applying Principal component analysis (PCA) to modify and reduce the features. PCA subsequently enhances the important temporal characteristics identified by the LSTM network. The parameters of the KNN classifier were confirmed by using fivefold cross-validation after making hyperparameter adjustments. The evaluation of the proposed model involved the use of four datasets: 1) telemetry data from the tested IoT network (TON-IoT), 2) Edith Cowan University-Internet of Health Things (ECU-IoHT) dataset, 3) intensive care unit (ICU) dataset, and 4) Washington University in St. Louis Enhanced Healthcare Surveillance System (WUSTL-EHMS) dataset. The proposed model achieved 99.9% accuracy, recall, F1 score, and precision on the WUSTL-EHMS dataset. The proposed technique efficiently mitigates cyber threats in healthcare environments.
Reliable decision tree model to detect Botnet traffic in the Internet of Vehicles (IoV)
Researcher: Dr. Ali Assi
Machine learning algorithms have offered unprecedented solutions for many real-world problems. These algorithms frequently involve using a large number of features. However, in many real-world applications, several of the used features could be not very informative due to uncertainties in the data, such as noise and residual variation. Decision trees are among the most preferred classification models. This is due to their simplicity, explainability, and readability. However, inaccuracies in the data could impact the construction stage of decision trees and thus hinder their results. Feature selection and feature construction present promising research direction to enhance the performance of decision tree models. In this project, a novel strategy including both feature selection and feature construction could be defined. This strategy can be applied in multiple applications. Out of them, I am working on constructing a reliable decision tree model that can effectively detect Botnet traffic in the Internet of Vehicles (IoV).
Blockchain
Blockchain technology via the lens of smart contract and consensus protocols
Researcher: Dr. Ioannis Karamitsos
In this area, Dr. Ioannis studies the design and development of different platforms such as Ethereum and Hyperledger. Dr. Ioannis also designs smart contract conceptual model for different industries. Finally, he examines the post quantum security mechanisms for the blockchain area.
Metaverse
Metacities
Researcher: Dr. Ioannis Karamitsos
Dr. Ioannis is applying metaverse in different vertical industry sectors, especially the shift of the smart cities (real estate) to Metacities.
Mobility
Enhancing Mobile Localization with Visual-Semantic SLAM and Event Cameras for Robust Autonomous Navigation
Researcher: Dr. Jinane Mounsef
Collaborators: Lebanese American University, University of Sharjah, BITS Pilani Dubai
Simultaneous Localization and Mapping (SLAM) is a key technology for mobile robots and autonomous systems, allowing them to navigate and map their environment. Traditional SLAM methods, which rely on standard visual inputs, often face challenges in dynamic or low-feature environments. This research investigates the integration of visual and semantic SLAM techniques, along with the use of event cameras, to create a more robust mobile localization system. Event cameras, which capture pixel-level changes in intensity at high temporal resolutions, provide significant advantages in fast-moving and low-light scenarios. By combining the high temporal accuracy of event cameras with visual SLAM, which tracks a robot's movement, and semantic SLAM, which assigns meaningful labels to objects and scenes, the system achieves enhanced localization accuracy and resilience. The fusion of these techniques allows for more reliable mobile localization, even in complex and challenging environments, improving the robustness of autonomous navigation systems in both indoor and outdoor applications.
Communications
Using spiking neural networks (SNN) to optimize resource-scheduling for deploying network slices in 5G
Researcher: Dr. Omar Abdul Latif
In this ongoing project, we are utilizing SNN that provides low-power and event-driven features that fits perfectly with 5G network slicing requirements. The simulation results were published and good feedback was received. We are currently working on real-life implementation on 5G core that was acquired as part of the Digital Transformation Lab at RIT.
- Click here to view the paper - link 1
- Click here to view the paper - link 2
- Click here to view the paper - link 3
- Click here to view the paper - link 4
Enhancing Spectrum Utilization in 5G Private Networks Through AI-Driven Cognitive Radio Technology
Researcher: Dr. Omar Abdul Latif
The scarcity of available spectrum resources for 5G systems poses a significant challenge for the efficient operation of these networks. This research project aims to investigate the application of cognitive radio technology, which utilizes AI, for spectrum sharing in 5G private networks, with the goal of enhancing spectrum utilization, optimizing network performance, and enabling coexistence with other wireless systems.
Data Science
Graph theory mining on the generative adversarial networks (GANs) and on Generative AI
Researcher: Dr. Ioannis Karamitsos
Dr. Ioannis is working with Diffusion Models, on MLOps pipelines framework and the optimization of dynamic systems for Machine Learning.
Understanding the theory, algorithms, modeling and practical aspects
Researcher: Dr. Ioannis Karamitsos
Dr. Ioannis is working on producing entire ML pipelines improving the various components of large systems and producing high quality in the intersection of data science concepts and computation.
Unsupervised Instance Matching in Large-Scale Knowledge Graphs Using GANs and Shared-Latent Space Translation
Researcher: Dr. Ali Assi
The Instance Matching technique involves identifying instances across different Knowledge Graphs (KGs) that refer to the same real-world entity. This process typically requires significant time and effort, especially with large KGs. Traditionally, this problem is addressed in a supervised manner, where a set of corresponding instance pairs across different KGs is available. However, in real-world applications involving billions of instances, such sets are often incomplete, adversely affecting the recall score. To address this challenge, our proposal explores an unsupervised approach by representing the instance matching problem as a language translation task. This is achieved through the use of a shared-latent space based on Generative Adversarial Networks (GANs), which allows for effective matching even in the absence of complete paired instances.