Less Is More In Semiconductor Manufacturing
Reducing sensors lowers costs, improves reliability
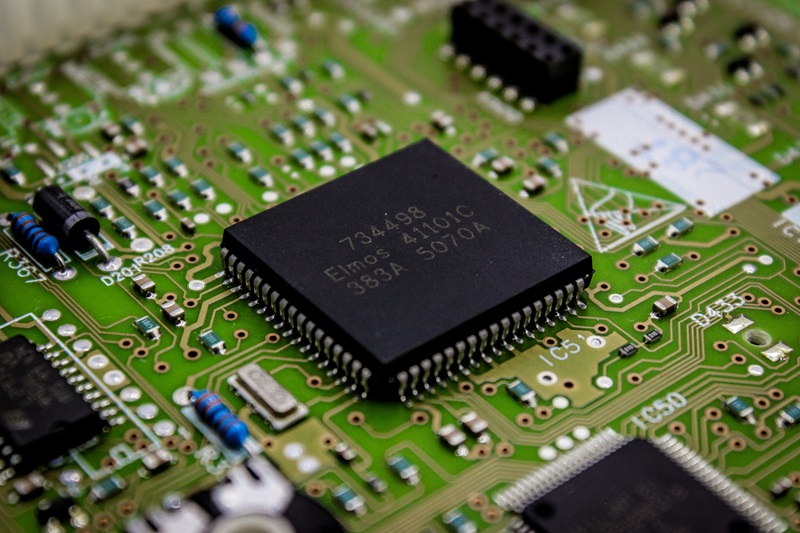
In the semiconductor industry, virtual metrology (VM), an efficient technique for monitoring quality improvement, generates a predictive model using real-time data from equipment sensors—for example, temperature, gas, and flow sensors—in conjunction with measured wafer quality characteristics. However, VM in wafer production processes usually employs multiple sensors, which leads to increased costs.
In response to this problem, Ali Tosyali, assistant professor in the department of MIS, marketing, and analytics, presents a virtual metrology method that reduces the number of sensors required for data collection during VM, thus providing benefits such as lower costs, high compactness, and improved reliability. Professor Tosyali along with his co-authors published this research in an article, “True sparse PCA [principal component analysis] for reducing the number of essential sensors in virtual metrology,” in International Journal of Production Research. To assess the performance of their VM method, Tosyali and his collaborators compared it with five well-established benchmark techniques. The results of their experiments indicate that the proposed method is effective in explaining a similar amount of total variance in the production process while utilizing a reduced number of variables.
While the focus in this article is on semiconductors, the proposed method is suitable for application throughout the field of VM, with the objective of decreasing the necessary number of sensors, thereby reducing both the initial and maintenance expenses associated with sensor acquisition as well as the overall cost of data processing.
View paper in International Journal of Production Research (May 2023), True sparse PCA for reducing the number of essential sensors in virtual metrology.